Prognostic Factors for Recurrence And Survival of Patients with Breast Cancer Using A Multi-state Model
A Multi state Model of Breast Cancer
DOI:
https://doi.org/10.31661/gmj.v13i.3043Keywords:
Multi-state Model; Survival Probability; Prognostic Factors; Breast CancerAbstract
Background: In many medical studies, patients may experience various events. The analysis in such studies is often administrated using multi-state models. The current study aimed to investigate the effect of risk factors and transition probability on recurrence and death in patients with breast cancer. Materials and Methods: This study was a retrospective cohort study on 814 women with breast cancer admitted to Shahid Ramezanzadeh Radiotherapy Center in Yazd province in Iran between the years 2004 -2012 and were followed until 2016. A multi-state model is applied for data analysis in the R 3.4.1 programming language. Results: Of the 814 patients, 40(5%) experienced recovery after initial treatment and 177(20.7%) experienced the death after initial treatment. For the first year, the transition probabilities from the initial treatment to recovery were estimated at 1.4%, to death was 17% and for recovery to death, it was 29%. The mean sojourn times were estimated as 2.93 and 9.8 years for the treatment and recovery, respectively. Conclusion: Multi-state models predict the transition probabilities in different states of disease, in addition, transition probabilities, mean sojourn time, and hazard ratio in each state can help physicians find suitable care for patients with breast cancer.
References
Sayed EN, Weber AS. editors Health information delivery outside the clinic in a developing nation: The Qatar Cancer Society in the State of Qatar. In Meeting Health Information Needs Outside Of Healthcare. 2015;1: 169-189.
https://doi.org/10.1016/B978-0-08-100248-3.00008-1
Farahani MV, Dizaji PA, Rashidi H, Mokarian F, Biglarian A. Application of Multi-State Model in Analyzing of Breast Cancer Data. J Res Health Sci. 2019; 19(4):e00465.
Marchetti MA, Codella NC, Dusza SW, Gutman DA, Helba B, Kalloo A, et al. Results of the 2016 International Skin Imaging Collaboration International Symposium on Biomedical Imaging challenge: Comparison of the accuracy of computer algorithms to dermatologists for the diagnosis of melanoma from dermoscopic images. J Am Acad Dermatol. 2018; 78(2):270-7.
https://doi.org/10.1016/j.jaad.2017.08.016
Parkin DM, Bray F, Ferlay J, Pisani P. Global cancer statistics, 2002. CA: CA Cancer J. 2005; 55(2):74-108.
https://doi.org/10.3322/canjclin.55.2.74
Siegel RL, Miller KD, Jemal A. Cancer statistics, 2015. CA: CA Cancer J. 2015; 65(1):5-29.
https://doi.org/10.3322/caac.21254
Amereh F, Jahangiri-Rad M, Mazloomi S, Rafiee M. The role of environmental and lifestyle factors in the incidence and prevalence of cancer. J Environ Health Sci Eng. 2017; 4(1):30-42.
https://doi.org/10.18869/acadpub.jehe.4.1.30
Harirchi I, Karbakhsh M, Kashefi A, Momtahen AJ. Breast cancer in Iran: results of a multi-center study. Asian Pac J Cancer Prev. 2004; 5(1):24-7.
Treska V, Cerna M, Liska V, Treskova I, Narsanska A, Bruha J. Surgery for breast cancer liver metastases-factors determining results. Anticancer Res. 2014; 34(3):1281-6.
Sessler DI, Pei L, Huang Y, Fleischmann E, Marhofer P, Kurz A, et al. Recurrence of breast cancer after regional or general anaesthesia: a randomised controlled trial. lancet. 2019; 394(10211):1807-1815.
https://doi.org/10.1016/S0140-6736(19)32313-X
Tanha J, Salarabadi H, Aznab M, Farahi A, Zoberi M. Relationship among prognostic indices of breast cancer using classification techniques. Inform Med Unlocked. 2020; 18:100265.
https://doi.org/10.1016/j.imu.2019.100265
Putter H, van der Hage J, de Bock GH, Elgalta R, van de Velde CJ. Estimation and prediction in a multi-state model for breast cancer. Biom J. 2006; 48(3):366-80.
https://doi.org/10.1002/bimj.200510218
Babaee E, Nafissi N, Tehrani-Banihashem A, Eshrati B, Janani L, Nojomi M. The effects of prognostic factors on metastasis and survival of patients with breast cancer using a multi-state model. Middle East J. Cancer.2021; 13(1):150-8.
https://doi.org/10.1016/S0960-9776(21)00194-6
Arab Borzu Z, Baghestani AR, Talebi Ghane E, Saeedi A, Akhavan A. Survival Analysis of Iranian Patients with Breast Cancer Using Joint Frailty Model with a Cure Rate. Middle East J. Cancer.2021; 12(2):228-34.
Hajihosseini M, Faradmal J, Sadighi-Pashaki A. Survival analysis of breast cancer patients after surgery with an intermediate event: application of illness-death model. Iran J Public Health. 2015; 44(12):1677.
Al-Saleh K, Salah T, Arafah M, Husain S, Al-Rikabi A, Abd El-Aziz N. Prognostic significance of estrogen, progesterone and HER2 receptors' status conversion following neoadjuvant chemotherapy in patients with locally advanced breast cancer: Results from a tertiary Cancer Center in Saudi Arabia. PLoS One. 2021; 16(3):e0247802.
https://doi.org/10.1371/journal.pone.0247802
Grover G, Swain PK, Goel K, Singh V. Multistate Markov modelling for disease progression of breast cancer patients based on CA15-3 marker. Thailand Statistician. 2018; 16(2):129-39.
Putter H, Fiocco M, Geskus RB. Tutorial in biostatistics: competing risks and multi-state models. Statistics in medicine. 2007; 26(11):2389-430.
https://doi.org/10.1002/sim.2712
Akbari M, Mozaffar M, Heidari A, Zirakzadeh H, Akbari A, Akbari M, et al. Recurrence and survival effect in breast conserving surgery: What are the predictive and/or prognostic factors. Iran J Cancer Prev. 2011; 4(2):49-54.
Hung M, Xu J, Nielson D, Bounsanga J, Gu Y, Hansen AR, et al. Evaluating the prediction of breast cancer survival using lymph node ratio. Iran J Cancer Prev. 2018; 21(3):315-20.
https://doi.org/10.4048/jbc.2018.21.e35
Yang Z-Y, Di M-Y, Yuan J-Q, Shen W-X, Zheng D-Y, Chen J-Z, et al. The prognostic value of phosphorylated Akt in breast cancer: a systematic review. Sci Rep. 2015; 5(1):1-9.
https://doi.org/10.1038/srep07758
Kanyılmaz G, Yavuz BB, Aktan M, Karaağaç M, Uyar M, Fındık S. Prognostic importance of Ki-67 in breast cancer and its relationship with other prognostic factors. Eur J Breast Health. 2019; 15(4):256.
https://doi.org/10.5152/ejbh.2019.4778
Meshkat M, Baghestani AR, Zayeri F, Khayamzadeh M, Akbari ME. Survival probability and prognostic factors of Iranian breast cancer patients using cure rate model. Breast J. 2018;24(6):1015-8.
https://doi.org/10.1111/tbj.13120
Wu S-G, He Z-Y, Li Q, Sun J-Y, Li F-Y, Lin Q, et al. Prognostic value of metastatic axillary lymph node ratio for Chinese breast cancer patients. PLoS One. 2013; 8(4):e61410.
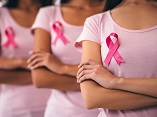
Published
How to Cite
Issue
Section
License
Copyright (c) 2024 Galen Medical Journal

This work is licensed under a Creative Commons Attribution 4.0 International License.