A Short Review on the Impact of Artificial Intelligence in Diagnosis Diseases: Role of Radiomics In Neuro-Oncology: Impact of AI in Neuro-Oncology Diseases
Impact of AI in Neuro-Oncology Diseases
DOI:
https://doi.org/10.31661/gmj.v12i.3158Keywords:
Artificial Intelligence, Early Diagnosis, Neouro-Oncology, Machine Learning, RadiomicsAbstract
Artificial Intelligence (AI) is rapidly transforming various aspects of healthcare, including the field of diagnostics and treatment of diseases. This review article aimed to provide an in-depth analysis of the impact of AI, especially, radiomics in the diagnosis of neuro-oncology diseases. Indeed, it is a multidimensional task that requires the integration of clinical assessment, neuroimaging techniques, and emerging technologies like AI and radiomics. The advancements in these fields have the potential to revolutionize the accuracy, efficiency, and personalized approach to diagnosing neuro-oncology diseases, leading to improved patient outcomes and enhanced overall neurologic care. However, AI has some limitations, and ethical challenges should be addressed via future research.
References
Nakamura H, Takami H, Yanagisawa T, Kumabe T, Fujimaki T, Arakawa Y, et al. The Japan Society for Neuro-Oncology guideline on the diagnosis and treatment of central nervous system germ cell tumors. Neuro Oncol. 2022;24(4):503-15.
https://doi.org/10.1093/neuonc/noab242
Shafizadeh M, Farzaneh F, Kankam SB, Jangholi E, Shafizadeh Y, Khoshnevisan A. Effects of postoperative intravenous Cyclosporine treatment on the survival and functional performance status of patients with glioblastoma: A randomized, triple-blinded, placebo-controlled clinical trial. World Neurosurg. 2023:176:e548-e56.
https://doi.org/10.1016/j.wneu.2023.05.101
Sturm D, Capper D, Andreiuolo F, Gessi M, Kölsche C, Reinhardt A, et al. Multiomic neuropathology improves diagnostic accuracy in pediatric neuro-oncology. Nat Med. 2023;29(4):917-26.
https://doi.org/10.1038/s41591-023-02255-1
Magge RS, Barbaro M, Fine HA. Innovations in neuro-oncology. World Neurosurg. 2021:151:386-91.
https://doi.org/10.1016/j.wneu.2021.02.093
Germann J, Zadeh G, Mansouri A, Kucharczyk W, Lozano AM, Boutet A. Untapped neuroimaging tools for neuro-oncology: connectomics and spatial transcriptomics. Cancers (Basel). 2022;14(3):464.
https://doi.org/10.3390/cancers14030464
Pasquini L, Peck KK, Jenabi M, Holodny A. Functional MRI in Neuro-Oncology: State of the Art and Future Directions. Radiology. 2023;308(3):e222028.
https://doi.org/10.1148/radiol.222028
Kaul V, Enslin S, Gross SA. History of artificial intelligence in medicine. Gastrointest Endosc. 2020;92(4):807-12.
https://doi.org/10.1016/j.gie.2020.06.040
Kumar Y, Koul A, Singla R, Ijaz MF. Artificial intelligence in disease diagnosis: a systematic literature review, synthesizing framework and future research agenda. J Ambient Intell Humaniz Comput. 2023;14(7):8459-86.
https://doi.org/10.1007/s12652-021-03612-z
Shen J, Zhang CJ, Jiang B, Chen J, Song J, Liu Z, et al. Artificial intelligence versus clinicians in disease diagnosis: systematic review. JMIR Med Inform. 2019;7(3):e10010.
Ao C, Jin S, Ding H, Zou Q, Yu L. Application and development of artificial intelligence and intelligent disease diagnosis. Curr Pharm Des. 2020;26(26):3069-75.
https://doi.org/10.2174/1381612826666200331091156
Lohmann P, Galldiks N, Kocher M, Heinzel A, Filss CP, Stegmayr C, et al. Radiomics in neuro-oncology: Basics, workflow, and applications. Methods. 2021;188:112-21.
https://doi.org/10.1016/j.ymeth.2020.06.003
Albalkhi I, Bhatia A, Lösch N, Goetti R, Mankad K. Current state of radiomics in pediatric neuro-oncology practice: a systematic review. Pediatr Radiol. 2023;53(10):2079-91.
https://doi.org/10.1007/s00247-023-05679-6
Ehret F, Kaul D, Clusmann H, Delev D, Kernbach JM. Machine learning-based radiomics in neuro-oncology. Acta Neurochir Suppl. 2022:134:139-51.
https://doi.org/10.1007/978-3-030-85292-4_18
Aneja S, Chang E, Omuro A. Applications of artificial intelligence in neuro-oncology. Curr Opin Neurol. 2019;32(6):850-6.
https://doi.org/10.1097/WCO.0000000000000761
Di Nunno V, Fordellone M, Minniti G, Asioli S, Conti A, Mazzatenta D, et al. Machine learning in neuro-oncology: Toward novel development fields. J Neurooncol. 2022;159(2):333-46.
https://doi.org/10.1007/s11060-022-04068-7
Jin L, Shi F, Chun Q, Chen H, Ma Y, Wu S, et al. Artificial intelligence neuropathologist for glioma classification using deep learning on hematoxylin and eosin stained slide images and molecular markers. Neuro Oncol. 2021;23(1):44-52.
https://doi.org/10.1093/neuonc/noaa163
Ak M, Toll SA, Hein KZ, Colen RR, Khatua S. Evolving role and translation of radiomics and radiogenomics in adult and pediatric neuro-oncology. American Journal of Neuroradiology. 2022 Jun 1;43(6):792-801.
https://doi.org/10.3174/ajnr.A7297
Park JE, Kickingereder P, Kim HS. Radiomics and deep learning from research to clinical workflow: neuro-oncologic imaging. Korean J Radiol. 2020;21(10):1126-37.
https://doi.org/10.3348/kjr.2019.0847
Park JE, Kim HS, Kim D, Park SY, Kim JY, Cho SJ, et al. A systematic review reporting quality of radiomics research in neuro-oncology: toward clinical utility and quality improvement using high-dimensional imaging features. BMC Cancer. 2020;20(1):29.
https://doi.org/10.1186/s12885-019-6504-5
Bhatia A, Birger M, Veeraraghavan H, Um H, Tixier F, McKenney AS, et al. MRI radiomic features are associated with survival in melanoma brain metastases treated with immune checkpoint inhibitors. Neuro Oncol. 2019;21(12):1578-86.
https://doi.org/10.1093/neuonc/noz141
Fathi Kazerooni A, Bagley SJ, Akbari H, Saxena S, Bagheri S, Guo J, et al. Applications of radiomics and radiogenomics in high-grade gliomas in the era of precision medicine. Cancers (Basel). 2021;13(23):5921.
https://doi.org/10.3390/cancers13235921
Park JE, Kim HS. Radiomics as a quantitative imaging biomarker: practical considerations and the current standpoint in neuro-oncologic studies. Nucl Med Mol Imaging. 2018;52(2):99-108.
https://doi.org/10.1007/s13139-017-0512-7
Di Stefano AL, Picca A, Saragoussi E, Bielle F, Ducray F, Villa C, et al. Clinical, molecular, and radiomic profile of gliomas with FGFR3-TACC3 fusions. Neuro Oncol. 2020;22(11):1614-24.
https://doi.org/10.1093/neuonc/noaa121
Artzi M, Bressler I, Ben Bashat D. Differentiation between glioblastoma, brain metastasis and subtypes using radiomics analysis. J Magn Reson Imaging. 2019;50(2):519-28.
https://doi.org/10.1002/jmri.26643
Grommes C, Nayak L, Tun HW, Batchelor TT. Introduction of novel agents in the treatment of primary CNS lymphoma. Neuro Oncol. 2019;21(3):306-13.
https://doi.org/10.1093/neuonc/noy193
Nguyen AV, Blears EE, Ross E, Lall RR, Ortega-Barnett J. Machine learning applications for the differentiation of primary central nervous system lymphoma from glioblastoma on imaging: a systematic review and meta-analysis. Neurosurg Focus. 2018;45(5):E5.
https://doi.org/10.3171/2018.8.FOCUS18325
Verma G, Mohan S, Nasrallah MP, Brem S, Lee JY, Chawla S, et al. Non-invasive detection of 2-hydroxyglutarate in IDH-mutated gliomas using two-dimensional localized correlation spectroscopy (2D L-COSY) at 7 Tesla. J Transl Med. 2016;14(1):274.
https://doi.org/10.1186/s12967-016-1035-1
Zlochower A, Chow DS, Chang P, Khatri D, Boockvar JA, Filippi CG. Deep learning AI applications in the imaging of glioma. Top Magn Reson Imaging. 2020;29(2):115-21.
https://doi.org/10.1097/RMR.0000000000000237
Brady AP, Neri E. Artificial intelligence in radiology-ethical considerations. Diagnostics (Basel). 2020;10(4):231.
https://doi.org/10.3390/diagnostics10040231
Lillywhite A, Wolbring G. Coverage of ethics within the artificial intelligence and machine learning academic literature: The case of disabled people. Assist Technol. 2021;33(3):129-35.
https://doi.org/10.1080/10400435.2019.1593259
Taha B, Boley D, Sun J, Chen C. Potential and limitations of radiomics in neuro-oncology. J Clin Neurosci. 2021:90:206-11.
https://doi.org/10.1016/j.jocn.2021.05.015
Guan J. Artificial intelligence in healthcare and medicine: promises, ethical challenges and governance. Chin Med Sci J. 2019;34(2):76-83.
Rubinger L, Gazendam A, Ekhtiari S, Bhandari M. Machine learning and artificial intelligence in research and healthcare. Injury. 2023:54 (Suppl 3):S69-S73.
https://doi.org/10.1016/j.injury.2022.01.046
Vollmer S, Mateen BA, Bohner G, Király FJ, Ghani R, Jonsson P, et al. Machine learning and artificial intelligence research for patient benefit: 20 critical questions on transparency, replicability, ethics, and effectiveness. BMJ. 2020:368:l6927.
https://doi.org/10.1136/bmj.l6927
Sunarti S, Rahman FF, Naufal M, Risky M, Febriyanto K, Masnina R. Artificial intelligence in healthcare: opportunities and risk for future. Gac Sanit. 2021:35 (Suppl 1):S67-S70.
https://doi.org/10.1016/j.gaceta.2020.12.019
Jarvis T, Thornburg D, Rebecca AM, Teven CM. Artificial intelligence in plastic surgery: current applications, future directions, and ethical implications. Plast Reconstr Surg Glob Open. 2020;8(10):e3200.
https://doi.org/10.1097/GOX.0000000000003200
Pandey B, Mishra RB. Knowledge and intelligent computing system in medicine. Comput Biol Med. 2009;39(3):215-30.
https://doi.org/10.1016/j.compbiomed.2008.12.008
Kelly CJ, Karthikesalingam A, Suleyman M, Corrado G, King D. Key challenges for delivering clinical impact with artificial intelligence. BMC Med. 2019;17(1):195.
https://doi.org/10.1186/s12916-019-1426-2
Jain S, Naicker D, Raj R, Patel V, Hu YC, Srinivasan K, et al. Computational Intelligence in Cancer Diagnostics: A Contemporary Review of Smart Phone Apps, Current Problems, and Future Research Potentials. Diagnostics (Basel). 2023;13(9):1563.
https://doi.org/10.3390/diagnostics13091563
Rauschert S, Raubenheimer K, Melton PE, Huang RC. Machine learning and clinical epigenetics: a review of challenges for diagnosis and classification. Clin Epigenetics. 2020;12(1):51.
https://doi.org/10.1186/s13148-020-00842-4
Martin-Isla C, Campello VM, Izquierdo C, Raisi-Estabragh Z, Baeßler B, Petersen SE, et al. Image-Based Cardiac Diagnosis With Machine Learning: A Review. Front Cardiovasc Med. 2020; 7: 1.
https://doi.org/10.3389/fcvm.2020.00001
Prevedello LM, Halabi SS, Shih G, Wu CC, Kohli MD, Chokshi FH, et al. Challenges related to artificial intelligence research in medical imaging and the importance of image analysis competitions. Radiol Artif Intell. 2019;1(1):e180031.
https://doi.org/10.1148/ryai.2019180031
Brunasso L, Ferini G, Bonosi L, Costanzo R, Musso S, Benigno UE, et al. A spotlight on the role of radiomics and machine-learning applications in the management of intracranial meningiomas: a new perspective in neuro-oncology: a review. Life (Basel). 2022;12(4):586.
https://doi.org/10.3390/life12040586
Qiu Q, Duan J, Yin Y. Radiomics in radiotherapy: applications and future challenges. . Prec Radiat Oncol. 2020; 4: 29-33.
https://doi.org/10.1002/pro6.1087
Thompson RF, Valdes G, Fuller CD, Carpenter CM, Morin O, Aneja S, et al. Artificial intelligence in radiation oncology: a specialty-wide disruptive transformation?. Radiother Oncol. 2018;129(3):421-6.
https://doi.org/10.1016/j.radonc.2018.05.030
Lohmann P, Bousabarah K, Hoevels M, Treuer H. Radiomics in radiation oncology-basics, methods, and limitations. Strahlenther Onkol. 2020;196(10):848-55.
https://doi.org/10.1007/s00066-020-01663-3
Yip SS, Aerts HJ. Applications and limitations of radiomics. Phys Med Biol. 2016;61(13):R150-66.
https://doi.org/10.1088/0031-9155/61/13/R150
Peeken JC, Wiestler B, Combs SE. Image-guided radiooncology: the potential of radiomics in clinical application. Recent Results Cancer Res. 2020:216:773-94.
https://doi.org/10.1007/978-3-030-42618-7_24
Priya S, Liu Y, Ward C, Le NH, Soni N, Pillenahalli Maheshwarappa R, et al. Radiomic based machine learning performance for a three class problem in neuro-oncology: time to test the waters?. Cancers (Basel). 2021;13(11):2568.
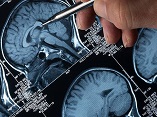
Published
How to Cite
Issue
Section
License
Copyright (c) 2023 Galen Medical Journal

This work is licensed under a Creative Commons Attribution 4.0 International License.