Machine Learning Approaches for Integrating Clinical and Radiographic Data in the Early Detection of Osteonecrosis of the Jaw
Machine Learning Approaches for Integrating Clinical Data in Osteonecrosis of the Jaw
DOI:
https://doi.org/10.31661/gmj.v13iSP1.3623Keywords:
Machine Learning; Radiographic Data; Osteonecrosis of the JawAbstract
Osteonecrosis of the jaw (ONJ) is a severe bone condition characterized by the progressive destruction of the jawbone, often associated with the long-term use of antiresorptive medications such as bisphosphonates. Early detection of ONJ remains a significant clinical challenge due to the subtle onset of symptoms and limitations in current diagnostic methods, which rely on clinical assessment and radiographic imaging. Conventional techniques, such as panoramic X-rays, computed tomography (CT), and magnetic resonance imaging (MRI), often fail to detect early-stage ONJ, delaying diagnosis until more advanced stages. Machine learning (ML) has emerged as a powerful tool for improving the early detection and diagnosis of ONJ by integrating clinical and radiographic data. ML algorithms, including supervised learning methods like random forests, support vector machines, and deep learning models such as convolutional neural networks, are particularly suited for analyzing complex datasets and identifying patterns that are undetectable by traditional methods. These models can enhance the sensitivity and specificity of ONJ detection, potentially leading to earlier interventions and improved patient outcomes. This paper reviews the current state of ML applications in ONJ detection, emphasizing the integration of clinical and radiographic data. It discusses various ML approaches, their potential to improve diagnostic accuracy, and the challenges involved in data integration. Also, this review highlights future directions of ML as a diagnostic tool that has the potential to revolutionize ONJ detection, offering a path toward earlier, more accurate diagnosis and better patient care.
References
VidalReal C, PerezSayans M, Suarez-Penaranda Jm, Gandara-Rey Jm, Garcia-Garcia A. Osteonecrosis of the jaws in 194 patients who have undergone intravenous bisphosphonate therapy in Spain. Med Oral Patol Oral Cirugia Bucal. 2015;20(3):e267-72.
https://doi.org/10.4317/medoral.20092
PMid:25662540 PMCid:PMC4464912
AlDhalaan NA, BaQais A, Al-Omar A. Medication-related Osteonecrosis of the Jaw: A Review. Cureus. 2020 Feb 10;12(2):e6944.
https://doi.org/10.7759/cureus.6944
PMid:32190495 PMCid:PMC7067354
Capocci M, Romeo U, Guerra F, Mannocci A, Tenore G, et al. Medication-related osteonecrosis of the jaws (MRONJ) and quality of life evaluation. a pilot study. 2017;168(4):e253-7.
Otto S, Pautke C, Hafner S, Hesse R, Reichardt LF, Mast G, et al. Pathologic Fractures in Bisphosphonate-Related Osteonecrosis of the Jaw-Review of the Literature and Review of Our Own Cases. Craniomaxillofacial Trauma Reconstr. 2013 Sep;6(3):147-54.
https://doi.org/10.1055/s-0033-1343776
PMid:24436752 PMCid:PMC3773037
Hamada H, Matsuo A, Koizumi T, Satomi T, Chikazu D. A simple evaluation method for early detection of bisphosphonate-related osteonecrosis of the mandible using computed tomography. J Cranio-Maxillofac Surg. 2014 Sep;42(6):924-9.
https://doi.org/10.1016/j.jcms.2014.01.012
PMid:24503386
Kizub DA, Miao J, Schubert MM, Paterson AHG, Clemons M, Dees EC, et al. Risk factors for bisphosphonate-associated osteonecrosis of the jaw in the prospective randomized trial of adjuvant bisphosphonates for early-stage breast cancer (SWOG 0307). Support Care Cancer. 2021 May;29(5):2509-17.
https://doi.org/10.1007/s00520-020-05748-8
PMid:32929540 PMCid:PMC7956914
Ghidini G, Manfredi M, Giovannacci I, Mergoni G, Sarraj A, Mureddu M, et al. Medication-related osteonecrosis of the jaw: risk factors in patients under biphosphonate versus patients under antiresorptive-antiangiogenic drugs. Minerva Dent Oral Sci. 2017 Jul;66(4):135-40.
https://doi.org/10.23736/S0026-4970.17.04056-0
PMid:28497661
Guerrero KGV, Garza NE, Silva JYG, Ruiz RR, Romero ADS, Cepeda MAAN, et al. Osteonecrosis of the jaw: An update. Int J Appl Dent Sci. 2021 Jul 1;7(3):173-7.
https://doi.org/10.22271/oral.2021.v7.i3c.1297
Simpione G, Caldas Rj, Soares Mq, Rubira-Bullen Ir, Santos Ps. Tomographic study of Jaw bone changes in patients with bisphosphonate-related osteonecrosis. J Clin Exp Dent. 2020;12(3):e285-90.
https://doi.org/10.4317/jced.56265
PMid:32190200 PMCid:PMC7071540
Gaêta‐Araujo H, Vanderhaeghen O, Vasconcelos KDF, Coucke W, Coropciuc R, et al. Osteomyelitis, osteoradionecrosis, or medication‐related osteonecrosis of the jaws Can CBCT enhance radiographic diagnosis. Oral Dis. 2021 Mar;27(2):312-9.
https://doi.org/10.1111/odi.13534
PMid:32623770
Wongratwanich P, Shimabukuro K, Konishi M, Nagasaki T, Ohtsuka M, et al. Do various imaging modalities provide potential early detection and diagnosis of medication-related osteonecrosis of the jaw A review. Dentomaxillofacial Radiol. 2021 Sep 1;50(6):20200417.
https://doi.org/10.1259/dmfr.20200417
PMid:33411572 PMCid:PMC8404513
Fleisher KE, Raad RA, Rakheja R, Gupta V, Chan KC, Friedman KP, et al. Fluorodeoxyglucose Positron Emission Tomography With Computed Tomography Detects Greater Metabolic Changes That Are Not Represented by Plain Radiography for Patients With Osteonecrosis of the Jaw. J Oral Maxillofac Surg. 2014 Oct;72(10):1957-65.
https://doi.org/10.1016/j.joms.2014.04.017
PMid:25053572
Kwack DW, Park SM. Prediction of medication-related osteonecrosis of the jaw (MRONJ) using automated machine learning in patients with osteoporosis associated with dental extraction and implantation: a retrospective study. J Korean Assoc Oral Maxillofac Surg. 2023 Jun 30;49(3):135-41.
https://doi.org/10.5125/jkaoms.2023.49.3.135
PMid:37394932 PMCid:PMC10318313
Gürses BO, Alpoz E, Şener M, Çankaya H, Boyacıoğlu H, Güneri P. A support vector machine-based algorithm to identify bisphosphonate-related osteonecrosis throughout the mandibular bone by using cone beam computerized tomography images. Dentomaxillofacial Radiol. 2023 Apr;52(4):20220390.
https://doi.org/10.1259/dmfr.20220390
PMid:36988116 PMCid:PMC10170169
Matthies L, Gebrekidan MT, Braeuer AS, Friedrich RE, Stelzle F, Schmidt C, et al. Raman spectroscopy and U ‐Net deep neural network in antiresorptive drug‐related osteonecrosis of the jaw. Oral Dis. 2024 May;30(4):2439-52.
https://doi.org/10.1111/odi.14721
PMid:37650266
Choi SY, Kim JW, Oh SH, Cheon S, Yee J, Kim SJ, et al. Prediction of medication-related osteonecrosis of the jaws using machine learning methods from estrogen receptor 1 polymorphisms and clinical information. Front Med. 2023 Jun 21;10:1140620.
https://doi.org/10.3389/fmed.2023.1140620
PMid:37415765 PMCid:PMC10321771
Berg BI, Mueller A, Augello M, Berg S, Jaquiéry C. Imaging in Patients with Bisphosphonate-Associated Osteonecrosis of the Jaws (MRONJ). Dent J. 2016 Sep 2;4(3):29.
https://doi.org/10.3390/dj4030029
PMid:29563471 PMCid:PMC5806936
Avril L, Lombardi T, Ailianou A, Burkhardt K, Varoquaux A, Scolozzi P, et al. Radiolucent lesions of the mandible: a pattern-based approach to diagnosis. Insights Imaging. 2014 Feb;5(1):85-101.
https://doi.org/10.1007/s13244-013-0298-9
PMid:24323536 PMCid:PMC3948901
Elhalawani H, Lin TA, Volpe S, Mohamed ASR, White AL, Zafereo J, et al. Machine Learning Applications in Head and Neck Radiation Oncology: Lessons From Open-Source Radiomics Challenges. Front Oncol. 2018 Aug 17;8:294.
Khader F, Müller-Franzes G, Wang T, Han T, Tayebi Arasteh S, Haarburger C, et al. Multimodal Deep Learning for Integrating Chest Radiographs and Clinical Parameters: A Case for Transformers. Radiology. 2023 Oct 1;309(1):e230806.
https://doi.org/10.1148/radiol.230806
PMid:37787671
Dinsdale NK, Bluemke E, Sundaresan V, Jenkinson M, Smith SM, Namburete AIL. Challenges for machine learning in clinical translation of big data imaging studies. Neuron. 2022 Dec;110(23):3866-81.
https://doi.org/10.1016/j.neuron.2022.09.012
PMid:36220099
Jovel J, Greiner R. An Introduction to Machine Learning Approaches for Biomedical Research. Front Med. 2021 Dec 16;8:771607.
https://doi.org/10.3389/fmed.2021.771607
PMid:34977072 PMCid:PMC8716730
Breiman L. Random Forests. Mach Learn. 2001;45(1):5-32.
https://doi.org/10.1023/A:1010933404324
Kim DW, Kim H, Nam W, Kim HJ, Cha IH. Machine learning to predict the occurrence of bisphosphonate-related osteonecrosis of the jaw associated with dental extraction: A preliminary report. Bone. 2018 Nov;116:207-14.
https://doi.org/10.1016/j.bone.2018.04.020
PMid:29698784
De Boves Harrington P. Support Vector Machine Classification Trees. Anal Chem. 2015 Nov 3;87(21):11065-71.
https://doi.org/10.1021/acs.analchem.5b03113
PMid:26461495
Bejani MM, Ghatee M. A systematic review on overfitting control in shallow and deep neural networks. Artif Intell Rev. 2021 Dec;54(8):6391-438.
https://doi.org/10.1007/s10462-021-09975-1
Kim JK, Choi GS, Kwak SY, Chang MC. Convolutional Neural Network Algorithm Trained with Anteroposterior Radiographs to Diagnose Pre-Collapse Osteonecrosis of the Femoral Head. Appl Sci. 2022 Sep 24;12(19):9606.
https://doi.org/10.3390/app12199606
Huynh PH, Nguyen VH, Do TN. A coupling support vector machines with the feature learning of deep convolutional neural networks for classifying microarray gene expression data. Modern approaches for intelligent information and database systems. 2018:233-43.
https://doi.org/10.1007/978-3-319-76081-0_20
Glielmo A, Husic BE, Rodriguez A, Clementi C, Noé F, Laio A. Unsupervised Learning Methods for Molecular Simulation Data. Chem Rev. 2021 Aug 25;121(16):9722-58.
https://doi.org/10.1021/acs.chemrev.0c01195
PMid:33945269 PMCid:PMC8391792
Sinaga KP, Yang MS. Unsupervised K-Means Clustering Algorithm. IEEE Access. 2020;8:80716-27.
https://doi.org/10.1109/ACCESS.2020.2988796
Aggarwal R, Sounderajah V, Martin G, Ting DSW, Karthikesalingam A, King D, et al. Diagnostic accuracy of deep learning in medical imaging: a systematic review and meta-analysis. Npj Digit Med. 2021 Apr 7;4(1):65.
https://doi.org/10.1038/s41746-021-00438-z
PMid:33828217 PMCid:PMC8027892
Schwendicke F, Golla T, Dreher M, Krois J. Convolutional neural networks for dental image diagnostics: A scoping review. J Dent. 2019 Dec;91:103226.
https://doi.org/10.1016/j.jdent.2019.103226
PMid:31704386
Ishimura E, Zhu W, Marin E, Honma T, Sugano N, Ando W, et al. PCA-Assisted Raman Analysis of Osteonecrotic Human Femoral Heads. Methods Protoc. 2022 Jan 17;5(1):10.
https://doi.org/10.3390/mps5010010
PMid:35076564 PMCid:PMC8788499
Guo Z, Li X, Huang H, Guo N, Li Q. Deep Learning-Based Image Segmentation on Multimodal Medical Imaging. IEEE Trans Radiat Plasma Med Sci. 2019 Mar;3(2):162-9.
https://doi.org/10.1109/TRPMS.2018.2890359
PMid:34722958 PMCid:PMC8553020
von Rueden L, Mayer S, Sifa R, Bauckhage C, Garcke J. Combining machine learning and simulation to a hybrid modelling approach: Current and future directions. InAdvances in Intelligent Data Analysis XVIII: 18th International Symposium on Intelligent Data Analysis, IDA 2020, Konstanz, Germany, April 27-29, 2020, Proceedings 18 2020 (pp. 548-560). Springer International Publishing.
https://doi.org/10.1007/978-3-030-44584-3_43
Zhu W, Zhang X, Fang S, Wang B, Zhu C. Deep Learning Improves Osteonecrosis Prediction of Femoral Head After Internal Fixation Using Hybrid Patient and Radiograph Variables. Front Med. 2020 Oct 7;7(0):573522.
https://doi.org/10.3389/fmed.2020.573522
PMid:33117834 PMCid:PMC7575786
Sagi O, Rokach L. Ensemble learning: A survey. WIREs Data Min Knowl Discov. 2018 Jul;8(4):e1249.
https://doi.org/10.1002/widm.1249
Kini J, Fleischer S, Dave I, Shah M. Ensemble Modeling for Multimodal Visual Action Recognition [Internet]. arXiv preprint. 2023;2: 230805430.
Reber B, Van Dijk L, Anderson B, Mohamed ASR, Fuller C, Lai S, et al. Comparison of Machine-Learning and Deep-Learning Methods for the Prediction of Osteoradionecrosis Resulting From Head and Neck Cancer Radiation Therapy. Adv Radiat Oncol. 2023 Jul;8(4):101163.
https://doi.org/10.1016/j.adro.2022.101163
PMid:36798732 PMCid:PMC9926206
Ariji Y, Yanashita Y, Kutsuna S, Muramatsu C, Fukuda M, Kise Y, et al. Automatic detection and classification of radiolucent lesions in the mandible on panoramic radiographs using a deep learning object detection technique. Oral Surg Oral Med Oral Pathol Oral Radiol. 2019 Oct;128(4):424-30.
https://doi.org/10.1016/j.oooo.2019.05.014
PMid:31320299
Humbert-Vidan L, Patel V, Oksuz I, King AP, Guerrero Urbano T. Comparison of machine learning methods for prediction of osteoradionecrosis incidence in patients with head and neck cancer. Br J Radiol. 2021 Apr 1;94(1120):20200026.
https://doi.org/10.1259/bjr.20200026
PMid:33684314 PMCid:PMC8010531
Wang P, Liu X, Xu J, Li T, Sun W, Li Z, et al. Deep learning for diagnosing osteonecrosis of the femoral head based on magnetic resonance imaging. Comput Methods Programs Biomed. 2021 Sep;208:106229.
https://doi.org/10.1016/j.cmpb.2021.106229
PMid:34153870
Gong W, Cao L, Zhu Y, Zuo F, He X, Zhou H. Federated Inverse Reinforcement Learning for Smart ICUs With Differential Privacy. IEEE Internet Things J. 2023 Nov 1;10(21):19117-24.
https://doi.org/10.1109/JIOT.2023.3281347
Li T, Sahu AK, Talwalkar A, Smith V. Federated Learning: Challenges, Methods, and Future Directions. IEEE Signal Process Mag. 2020 May;37(3):50-60.
https://doi.org/10.1109/MSP.2020.2975749
Liu S, See KC, Ngiam KY, Celi LA, Sun X, Feng M. Reinforcement Learning for Clinical Decision Support in Critical Care: Comprehensive Review. J Med Internet Res. 2020 Jul 20;22(7):e18477.
PMid:32706670 PMCid:PMC7400046
Ernst D, Louette A. Introduction to reinforcement learning. Feuerriegel Hartmann J Janiesch C Zschech P2024 Gener Ai Bus Inf Syst Eng. 2024;66(1):111-26.
https://doi.org/10.1007/s12599-023-00834-7
Magudia K, Bridge CP, Andriole KP, Rosenthal MH. The Trials and Tribulations of Assembling Large Medical Imaging Datasets for Machine Learning Applications. J Digit Imaging. 2021 Dec;34(6):1424-9.
https://doi.org/10.1007/s10278-021-00505-7
PMid:34608591 PMCid:PMC8669054
Zhu L, Han J, Guo R, Wu D, Wei Q, Chai W, et al. An Automatic Classification of the Early Osteonecrosis of Femoral Head with Deep Learning. Curr Med Imaging Former Curr Med Imaging Rev. 2021 Jan 12;16(10):1323-31.
https://doi.org/10.2174/1573405615666191212104639
PMid:33461446
Sheller MJ, Edwards B, Reina GA, Martin J, Pati S, Kotrotsou A, et al. Federated learning in medicine: facilitating multi-institutional collaborations without sharing patient data. Sci Rep. 2020 Jul 28;10(1):12598.
https://doi.org/10.1038/s41598-020-69250-1
PMid:32724046 PMCid:PMC7387485
Wongvibulsin S, Wu KC, Zeger SL. Improving Clinical Translation of Machine Learning Approaches Through Clinician-Tailored Visual Displays of Black Box Algorithms: Development and Validation. JMIR Med Inform. 2020 Jun 9;8(6):e15791.
PMid:32515746 PMCid:PMC7312245
Ulrich CM, Grady C, Demiris G, Richmond TS. The Competing Demands of Patient Privacy and Clinical Research. Ethics Hum Res. 2021 Jan;43(1):25-31.
https://doi.org/10.1002/eahr.500076
PMid:33463073
Wang Y, Su H, Zhang B, Hu X. Learning Reliable Visual Saliency For Model Explanations. IEEE Trans Multimed. 2020 Jul;22(7):1796-807.
https://doi.org/10.1109/TMM.2019.2949872
Park SH, Choi J, Byeon JS. Key Principles of Clinical Validation, Device Approval, and Insurance Coverage Decisions of Artificial Intelligence. Korean J Radiol. 2021;22(3):442.
https://doi.org/10.3348/kjr.2021.0048
PMid:33629545 PMCid:PMC7909857
Muehlematter UJ, Daniore P, Vokinger KN. Approval of artificial intelligence and machine learning-based medical devices in the USA and Europe (2015-20): a comparative analysis. Lancet Digit Health. 2021 Mar;3(3):e195-203.
https://doi.org/10.1016/S2589-7500(20)30292-2
PMid:33478929
Wang SM, Hogg HDJ, Sangvai D, Patel MR, Weissler EH, Kellogg KC, et al. Development and Integration of Machine Learning Algorithm to Identify Peripheral Arterial Disease: Multistakeholder Qualitative Study. JMIR Form Res. 2023 Sep 21;7:e43963.
PMid:37733427 PMCid:PMC10557008
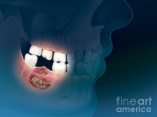
Published
How to Cite
Issue
Section
License
Copyright (c) 2024 Galen Medical Journal

This work is licensed under a Creative Commons Attribution 4.0 International License.